- How can we apply machine learning to asset management in the future?
- How do you ensure the robustness and reliability of your machine learning models when deployed in real-world asset management scenarios?
- In your experience, what are the main challenges when applying machine learning to financial time series and portfolio construction?
- What trends do you foresee as quantitative finance evolves, shaping the industry in the coming years?
- Fireside Chat
- How do you stay up-to-date with the latest advancements in machine learning and finance?
- How did you first become interested in quantitative finance and asset management?
- What advice would you give young professionals aspiring to pursue a career in quantitative finance and become successful asset managers?
What’s the Allure of Machine Learning in Trading? Creed&Bear’s Investment Head Shares Take
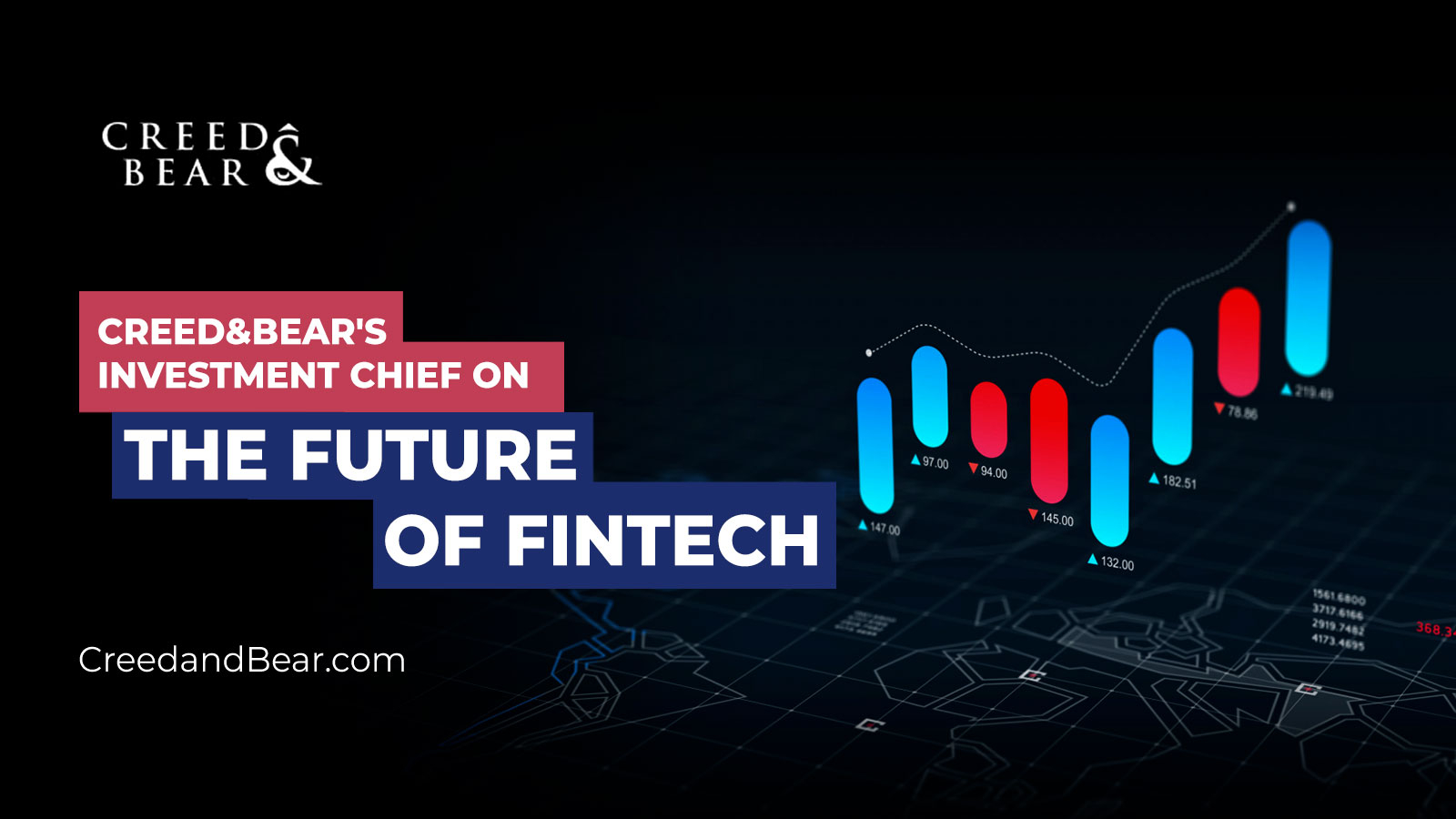
Disclaimer: The opinions expressed by our writers are their own and do not represent the views of U.Today. The financial and market information provided on U.Today is intended for informational purposes only. U.Today is not liable for any financial losses incurred while trading cryptocurrencies. Conduct your own research by contacting financial experts before making any investment decisions. We believe that all content is accurate as of the date of publication, but certain offers mentioned may no longer be available.
Artificial Intelligence (AI) and machine learning (ML) technologies will have the highest influence on the trading industry in the next three years, according to more than half of the respondents to a JPMorgan Chase survey of 835 institutional and professional traders.
Considering its fast-paced adoption in the financial world, we approached Andrea Nardon, Chief Investment Officer of Creed&Bear, a leading FinTech software company in the MENA region, to understand the applications of Machine Learning within the trading world, its future and what challenges adopters of AI and ML will face further down the road.
How can we apply machine learning to asset management in the future?
Applying machine learning techniques to financial time series to extract alpha is one of the most promising areas for traders everywhere. However, think about asset management as a whole. In that case, many, many areas are still controlled by individuals who are prone to mistakes. So, there are a lot of potential applications in such areas. For instance, you can use it in marketing. Today, lead generation is done by individuals, so you can use clustering models in unsupervised learning to help you identify where your next type of clients are.
How do you ensure the robustness and reliability of your machine learning models when deployed in real-world asset management scenarios?
This is when machine learning becomes almost an art. When you deploy machine learning in trading, you can use several tools to understand if the model has learned some input-output relationship or memorized that relationship.
There is no "special" recipe to answer this question. In whichever classification model you use, for instance, to classify the picture of an animal into a category, a cat into a cat box, or a dog into a dog box, you can easily understand from the output whether it has executed your instructions.
Today, with ChatGPT, the concept of generative AI looks almost magical. But how we communicate with it follows a very specific structure. And so once you get an outcome from ChatGPT, you immediately know whether that answer makes sense. For instance, when you apply ML to a financial time series for trading, the model tells you which asset to buy or sell. But the caveat is that there's no way to verify that. All you could do at that moment is to trust the model and wait and see if, over the time window it was trained, it produced the right outcome.
There are many techniques that we're following here at Creed&Bear to try to make sure that the model is well-trained and has learned. However, training ML models is more of an art than a science.
In your experience, what are the main challenges when applying machine learning to financial time series and portfolio construction?
The theoretical frameworks around portfolio construction go back to the 60s. We also have a long history with financial time series. We are now at a point where new researchers are taking these models forward to the next generation. But we're still at the inception of portfolio construction and machine learning.
The biggest challenge is not really on the technical side. It's relatively easy to apply these models to datasets. The challenge is understanding the outcome of these models. And it's very important that researchers understand the outcome.
Otherwise, the model ends up being a black box, and you need to accept the outcome as it is. The biggest challenge is opening the box and understanding the kind of input-output relationship the model has. Either memorize or learn over time. And if it isn't memorized, of course, that will lead to unstable results going forward.
It's very important that the researcher, who is myself in this case, can look through the model and see what the model has detected from the data samples provided.
What about when applying machine learning to cryptocurrencies as an asset class?
The biggest challenge for me was to understand the fundamental drivers of cryptocurrencies. In traditional assets, you have valuations, and people agree on valuation methods. But when it comes to crypto, how do you understand its value? What is driving the price of a coin up ten times, let's say, from a short period before?
I discovered a powerful behavioral element behind the cryptocurrency market, and so that is its biggest opportunity. At Creed&Bear, we gather inputs from different sources to understand how a specific coin behaves. And that forms the signal to generate trading strategies through machine learning models.
What trends do you foresee as quantitative finance evolves, shaping the industry in the coming years?
With all the technology we have at our disposal, I think it won't be very pleasant to see that the industry won't enjoy economies of scale in the future. I think asset managers will start being smaller. We've seen this already in the hedge fund space. Hedge funds that use many quantitative tools tend to run their operations tighter with a smaller number of people than you'd see in the traditional buy side.
The technology will lead to better products, more bespoke solutions, and more scalable products in general. Another trend I see is how advanced technology is going to tackle all things risk- and legal-related way more efficiently than what we're doing today.
Fireside Chat
How do you stay up-to-date with the latest advancements in machine learning and finance?
Because we're using machine learning and AI today, these models were invented just a few years ago. In fact, this is not true.
Most of the AI and machine learning models today were built decades ago. We can use them today simply because we now have the computing power that enables us to run these models.
So the evolution in terms of modeling has slowed down over the years. But the way to stay on top of it - not just from a theoretical point of view, but also from a practical point of view - is to catch up on what the academics have been publishing. And find out how researchers and PhD students apply these models to solve complex problems.
How did you first become interested in quantitative finance and asset management?
I remember this very well. I was 16 years old when my father and grandfather introduced me to the trading world. I got my hands on the balance sheet of a very famous Italian automobile company listed then in the Italian stock market. I remember computing some financial ratios, and very proudly, I discussed it with my father. Trying not to hurt my feelings, he asked whether I was aware that the balance sheet was only one aspect of the company's reality. From that moment on, I took a complete U-turn and nearly forgot about fundamentals for a long time. I just looked at quantitative methods to develop trading strategies.
What advice would you give young professionals aspiring to pursue a career in quantitative finance and become successful asset managers?
Become very strong computer scientists. Because today, with the kind of computing power we have, you can accomplish a lot. However, my tip would be to study the theoretical frameworks. We live in a world of big data. Researchers suggest that we have so many answers hidden in our datasets. In our case, that's trading data. But the reality is that you still need to know why the value of US Securities is at that level and why markets move up and down, so don't forget the theories.
About the Company
Founded in Zug, Switzerland in 2019, Creed&Bear is a FinTech company presently in Dubai actively researching and developing automated trading algorithms for asset managers, banks, and financial institutions. Creed&Bear specializes in providing bleeding-edge AI software solutions for trading digital assets.
To know more, follow the company on Twitter and LinkedIn, as well as on their website https://creedandbear.com/.
Related articles
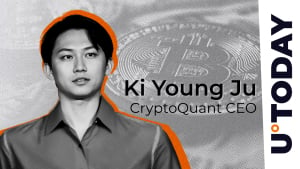
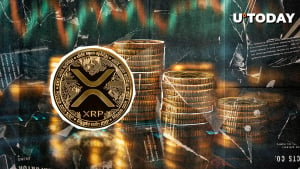